An Open-Source Knowledge Graph Ecosystem for the Life Sciences
Knowledge graphs (KGs) are used to integrate disparate data, decipher complex processes, and have frequently been used to systematically interrogate the biology underlying complicated systems and diseases. While it is clear that KGs are a useful structure for integrating complex biomedical data, their value within the Life Sciences has yet to be fully realized because: (1) existing open source KG construction methods are unable to account for the use of different vocabularies, are technically complex, difficult to use, and often scale poorly; (2) there are a lack of biologically and clinically meaningful benchmarks to evaluate KGs; and (3) privacy concerns when leveraging external data with patient-level information. To solve some of these KG construction challenges, we developed the PheKnowLator (Phenotype Knowledge Translator; https://github.com/callahantiff/PheKnowLator) Ecosystem, a suite of tools for constructing ontologically-grounded KGs built on FAIR Data Principles. Our presentation has two objectives. First, we will describe the PheKnowLator Ecosystem. Then, we will present results and lessons learned from two biomedical applications using PheKnowLator KGs. The first application demonstrates how a KG can be used to integrate clinical observations with publicly available biological experiments to infer unobserved patient-level molecular mechanisms. The second application describes our efforts to define meaningful heuristics to improve evidence-based recommendations for pharmacovigilance. We conclude by highlighting opportunities for KGs to solve real-world public health problems, such as automated adjudication and characterization of phenotypes, evidence-based feature selection for machine learning, and causal inference for pharmacovigilance.
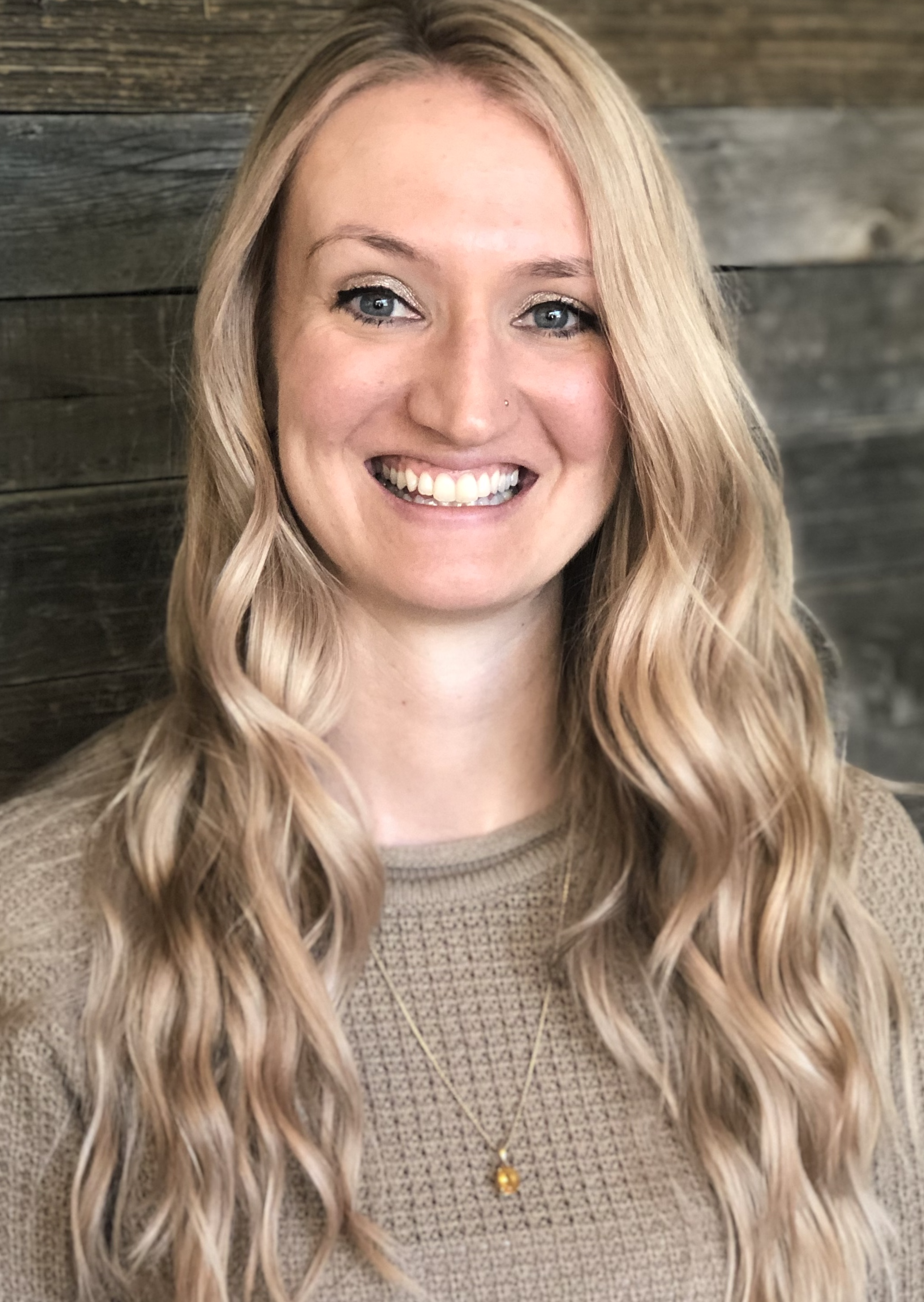