Building a knowledge graph of biomedical entities (genes, diseases, proteins, pathways) using a gene signature representing a particular disease condition or perturbation using half a million FAIR datasets
Knowledge graphs are powerful resources for the discovery of interactions and emergent properties in biological systems, ranging from single-cell to population level. Network approaches have been used numerous times to connect and amplify signals from individual genes, and have led to remarkable discoveries in biology, including drug discovery, protein function prediction, disease diagnosis, and precision medicine. We used transcriptomics data from GEO, Cbioportal, LINCS, TCGA and other public repositories to construct a knowledge graph of biomedical keywords (like gene names and disorders) from public literature abstracts (PubMed), for a biological condition/signature Hypoxia. We converted the knowledge of metadata (gene expression matrix) associated with each publication to a statistical score that can be used to link these biomedical keywords in the abstracts of the literature. Interpreted the results using a deep learning model to obtain genes that are either upregulated or downregulated by a master transcriptional regulator gene in that specific disease context (HIF1a gene for Hypoxia). The datasets were curated using transformer models tagging genes, disease, drugs, cell lines and cell types. Our method is first to use both data and metadata to construct a knowledge graph. To know more about our metadata curation refer to:https://fairtoolkit.pistoiaalliance.org/use-cases/fair-annotation-and-evaluation-of-rna-sequencing-and-microarray-data-elucidata/
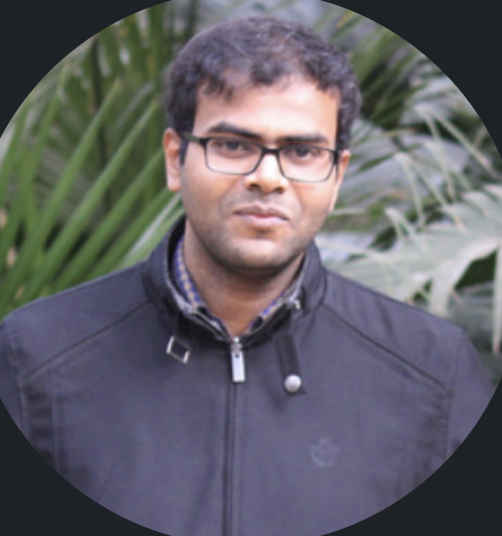