Biomedical Knowledge Graph: Build and Usage
Biomedical field has an abundance of well-maintained ontologies and knowledge sources for different sub-domains such as genomics and clinical trial. There is lot of value in being able to connect disparate but related sources of biomedical knowledge into a cohesive knowledge graph such as connecting adverse event data to clinical trial dataset for example. It also enables knowledge-driven query of the health data based on underlying terminology and it's relationships. For example, query for all patients with any form of diabetes could utilize the knowledge of diabetes concept and it's hierarchical relationships particularly child relationships to find all forms of diabetes in the knowledge base. A semantic biomedical knowledge graph will resolve biomedical concepts to a standard ontology or taxonomy and import relationships between those concepts. Moreover, it will also need to map concepts across component sources so that it can connect diverse sources of data. Another implication is that if biomedical NLP is used to resolve entity mentions in free-text, they will also need to resolve the entities to standard vocabulary or terminology. UMLS or Unified Medical Language System is used exactly for this. UMLS is a long-term project of the NLM (National Library of Medicine) which maintains mappings of concepts across various biomedical vocabularies, terminologies and taxonomies. The UMLS metathesaurus contains over 5 million plus terms organized by meaning into concepts and assigned a concept unique identifier or CUI. Abdominal Pain from our example earlier has codes "R10.9" and "21522001" in ICD10 and SNOMED respectively which will be mapped to UMLS CUI value of C0000737 in UMLS Metathesaurus thereby effectively mapping the concept of abdominal pain across the two different sources. As such, UMLS is a perfect candidate to build a semantic biomedical knowledge graph on top of. UMLS metathesaurus itself is not a vocabulary but it is an amalgam of over 200+ sources and vocabularies that are standards and thus helps to create mappings between these vocabularies and standards as well. A SKOS representation of UMLS concepts and relationships forms a baseline biomedical knowledge graph which can be augmented with other biomedical knowledge sources such as ClinicalTrials.gov dataset or CORD-19 dataset by resolving their concept mentions and extracted relationships to UMLS SKOS concepts. As mentioned earlier, a biomedical NLP tool which can resolve extracted concepts and relationships to UMLS SKOS concepts needs to be part of the tools repertoire. This talk will highlight such tools and methodologies used in constructing such a biomedical knowledge graph and some of it's potential usecases in clinical and analytics workflow.
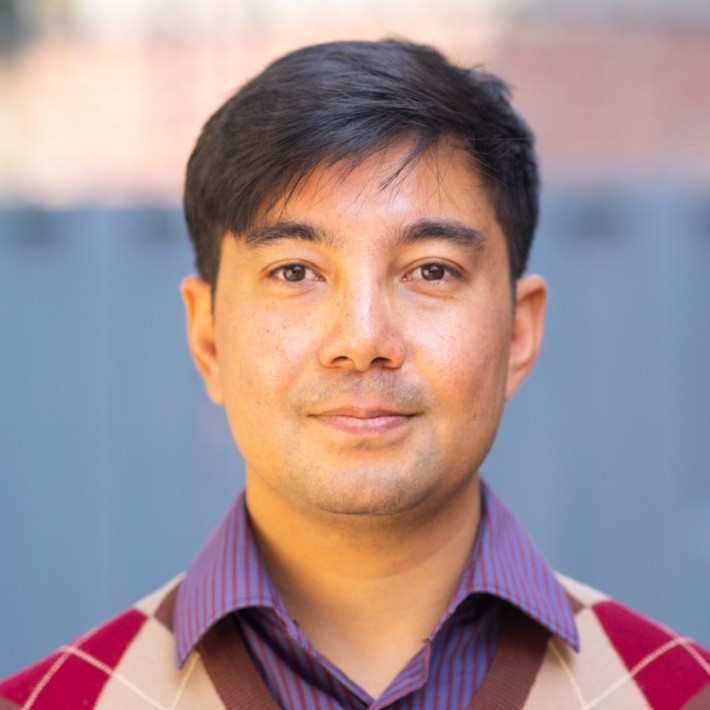