Data-Driven Discovery Science with FAIR Knowledge Graphs
The increasing availability of biomedical data offers tantalizing opportunities in support of computational discovery science. Yet, these data remain hard to find and to productively reuse because data and their metadata i) are wholly inaccessible, ii) are in non-standard or incompatible representations, iii) do not conform to community standards, and iv) iii) have unclear or highly restricted terms and conditions that preclude legitimate reuse. In this talk, I will discuss developments to create computational standards, platforms, and methods to wrangle knowledge into simple, but effective semantic and latent representations in support of biomedical knowledge discovery that are maximally FAIR (Findable, Accessible, Interoperable, and Reusable).
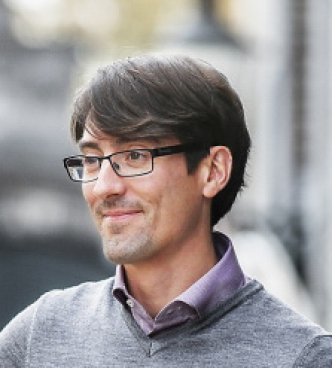
Michel Dumontier
Dr. Michel Dumontier is the Distinguished Professor of Data Science at Maastricht University and co-founder of the FAIR (Findable, Accessible, Interoperable and Reusable) data principles. His research focuses on the development of computational methods for scalable and responsible discovery science. Previously a faculty member at Carleton University in Ottawa and Stanford University in Palo Alto, Dr. Dumontier founded and directs the Institute of Data Science at Maastricht University. His work is supported through the Dutch National Research Agenda, the Netherlands Organization for Scientific Research, Horizon 2020/Europe, the European Open Science Cloud, the US National Institutes of Health, and a Marie-Curie Innovative Training Network. He is the editor-in-chief for the journal Data Science and is internationally recognized for his contributions in bioinformatics, biomedical informatics, and semantic technologies including ontologies and linked data.