Creating a Healthcare Knowledge Graph from Statistical Open Data
A variety of published open government data include multi-dimensional and statistical healthcare information, such as public health data and disease information which can be used in public services and provides social value to citizens [1][2]. The main purpose of the open government web portals is to allow individuals to efficiently access information, gain new insights, and make discoveries. Each statistical healthcare dataset usually has a metadata section and an observation section that includes the statistical observations. An observation includes dimensions and measures. For example, a record such as “number of ‘X’ disease is ‘Y’ in year ‘Z’” has X and Z as dimensions and Y as the metric. The health-related datasets in the current format in Canada's open government websites are isolated and cannot be queried. These sources are scattered in the government data portals, and users can access the information through specific searches in that data portal. The lack of meaning behind the statistical data makes it impossible to form a network and link this kind of data to infer, create and query knowledge [3]. Creating a knowledge graph by interconnecting the isolated healthcare datasets in open government data allows users to deduce relations and infer meaning. According to my previous research [4], there are 11 provinces and territories across Canada that publish around 11,771 datasets in different domains from the “Business and Economy” category to “Healthcare and Wellness”. They do not follow a unified standard or structure, and they are mostly published in different formats, including CSV, JSON, and Excel. A few allow users to export data in RDF format; however, they do not follow the Linked Data vocabulary standards such as RDF Cube vocabularies. The lack of a knowledge graph does not allow data consumers to answer questions like: "Which viral diseases had the most cases in a province in 2021?". A multi-dimensional structure should be followed consisting of measures (e.g., number of disease cases) and dimensions describing those measures (e.g., regions) to construct a healthcare knowledge graph over open statistical data. In this study, a statistical knowledge graph in healthcare was constructed based on the W3C standards by a) collecting a set of semi-structured statistical disease datasets from an open government data API, b) constructing an RDF knowledge graph based on a multi-dimensional model and transforming the data into the knowledge graph, c) interlinking disease names to an external ontology and similar datasets in other provinces, and d) storing the knowledge graph in a triple store (Jena). A set of semantic rules based on SWRL was also designed to incorporate and build semantic relationships. An external disease ontology (DOID) was used to enrich the created knowledge graph based on disease names. The super-classes of each disease were fetched from the disease ontology to add more semantics to statistical data. This additional semantics allows for designing more advanced queries against the knowledge graph, given that the disease categories were not available in the raw datasets. For example, to get the “list of the viral infectious diseases along with their number of cases in different years”, the reasoner can retrieve the answers using the parent-child relationship between disease names and their super-classes. The study shows integrating open statistical datasets in healthcare from multiple sources using ontologies and interlinking them potentially leads to valuable data sources and generates a dense knowledge graph with cross-dimensional information.
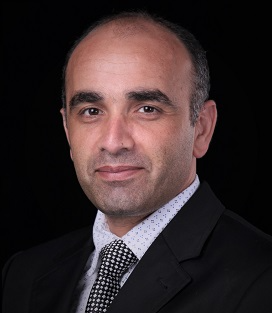