An automatically assembled knowledge graph from literature-extracted molecular biology knowledge with human-machine dialogue to support biomedical discovery
Understanding human biology in a healthy and diseased setting, and developing effective therapies crucially relies on knowledge of molecular biology mechanisms. However, this knowledge is distributed across millions of scientific publications as unstructured text and only some of it is readily available in structured databases. With around four thousand new publications appearing each day in biomedicine, keeping up with the rate of new findings in a systematic way is beyond human capacity. We developed INDRA, an automated knowledge assembly framework that integrates multiple text mining systems and biological pathway databases and standardizes statements representing relations between concepts extracted from these sources at scale. To guide knowledge assembly, INDRA uses an ontology that captures a wide range of concept types including genes, proteins, and their families and complexes, small molecules (e.g., drugs, metabolites), biological processes, diseases, etc. Through a process of knowledge assembly INDRA fixes certain systematic errors (e.g., using machine-learned disambiguation models to improve named entity normalization) and calculates a belief score associated with each statement taking into account all the evidence (from different papers or databases) supporting it. Having processed all publicly available literature content from the PubMed and PubMedCentral repositories with multiple text mining systems, and combining these extractions with the content of more than a dozen structured databases, we obtained 6.2 million normalized statements about molecular mechanisms with 18 million distinct evidences supporting them. Molecular mechanisms assembled by INDRA can be further enriched when extended with non-causal/non-mechanistic context describing properties (e.g., that a given protein is a kinase) and data (e.g., that a gene is expressed in a given cancer cell line). We therefore constructed a knowledge graph combining all INDRA statements with ontological relations, and relations representing properties and data, and made this available in a Neo4j instance as a labeled property graph called INDRA CoGEx (INDRA Context Graph Extension). The graph contains 54 million nodes and 325 million edges accounting for both the relations between relevant concepts and a representation of their supporting evidence. We demonstrate the utility of this graph by deriving a cancer-type-specific network and using it to rank potentially important molecular regulators upon perturbation. Finally, we connected INDRA CoGEx to a human-machine dialogue system called CLARE allowing gaining insights from the represented knowledge in an interactive fashion. CLARE interprets natural language questions and maps them to graph query templates to produce responses rendered as natural language with accompanying structured browsing. Notably, CLARE uses context-aware coreference resolution to support follow-up questions with respect to previously mentioned results allowing for powerful sequential knowledge exploration. CLARE is available as a Slack application and has been integrated into the workspaces of multiple biology research groups such as the COVID-19 Disease Map community.
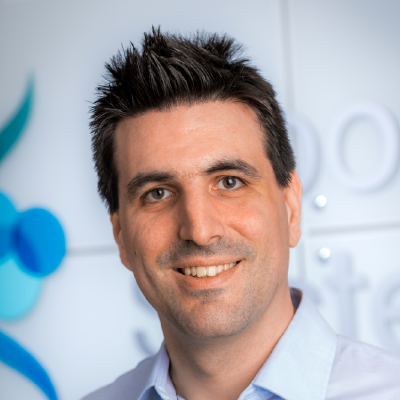